Thomas Nield
Random thoughts about analytics, business technology, and other musings.
YouTube Videos

A Simple Neural Network
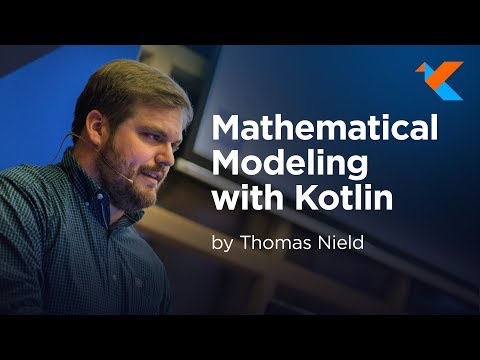
KotlinConf 2018 - Mathematical Modeling

Creating a Sudoku Solver from Scratch

Traveling Salesman Problem

Text Categorization w/ Naive Bayes

Monty Hall Problem
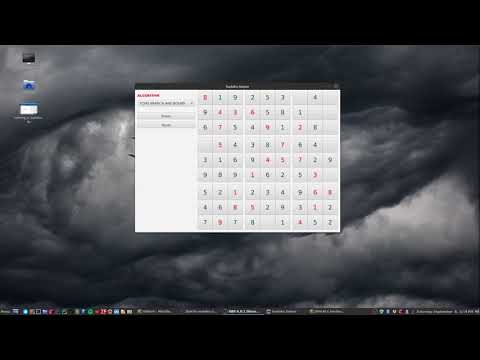
Solving World's Hardest Sudoku